Prova de Doutoramento do aluno Miguel Serras Vasco
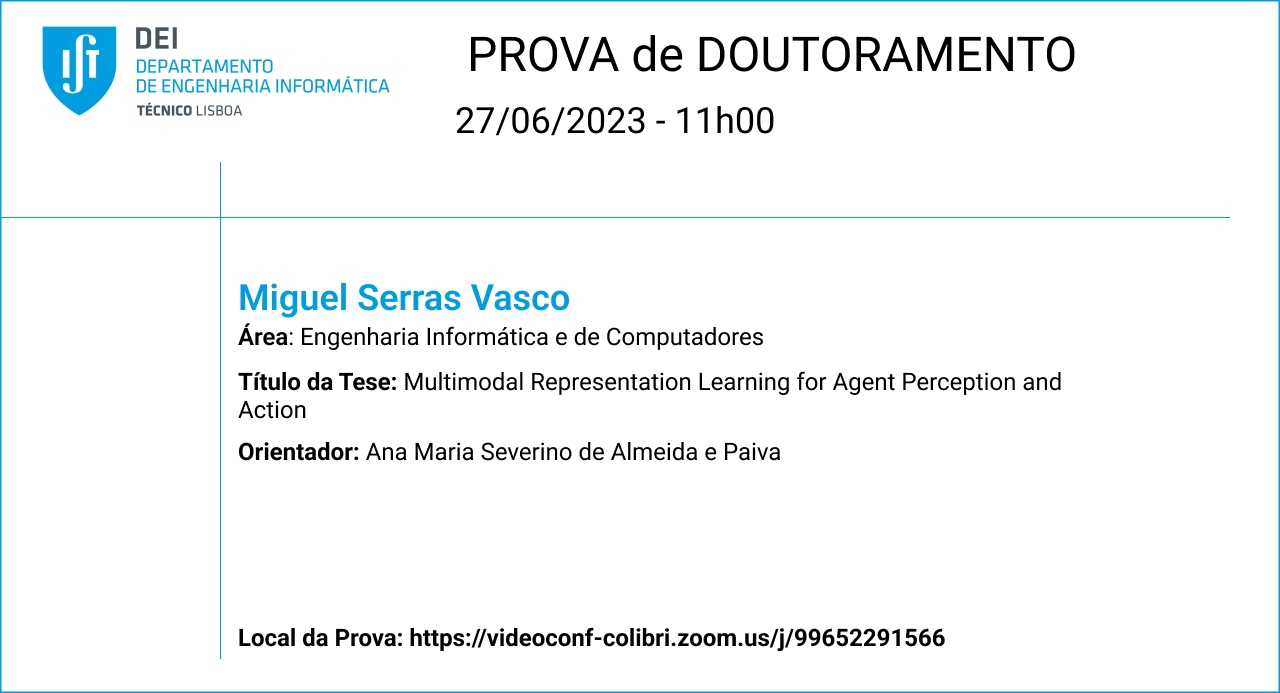
Área: Engenharia Informática e de Computadores
Título da Tese: Multimodal Representation Learning for Agent Perception and Action
Local da Prova: https://videoconf-colibri.zoom.us/j/99652291566
Data: 27/06/2023
Hora: 11h00
Abstract: In this thesis, we address the problem of endowing agents with mechanisms to learn multimodal representations from sensory data and to allow the execution of tasks under partial perceptual availability, i.e., considering different subsets of available perceptions. We explore learning multimodal representations from supervised, unsupervised, and self-supervised approaches and then leverage such representations for reinforcement learning tasks under changing conditions of perceptual availability at execution time. In the context of supervised representation learning, we contribute a novel multimodal representation of human actions and a learning algorithm that enables agents to consider contextual information provided in action demonstrations, allowing sample-efficient recognition of human actions. In the context of unsupervised representation learning, we explore the cross-modality inference problem - the estimation of missing perceptual data from available perceptions - and contribute a novel hierarchical multimodal generative model that addresses the requirements of computational cross-modality generation. In the context of self-supervised representation learning, we propose a novel framework based on multimodal contrastive learning that provides robust performance to downstream tasks with missing modality information at test time. Furthermore, we introduce multimodal policy transfer in reinforcement learning, where an agent must learn and exploit policies over different subsets of input modalities and instantiate such problem in the context of Atari Games. Finally, we extend our ideas of multimodal perceptual models to multi-agent settings and introduce the paradigm of hybrid execution for multi-agent reinforcement learning, allowing agents to perform cooperative tasks across all possible communication levels in the environment while exploiting passively shared information at execution time.