Prova de Doutoramento do aluno Miguel Afonso Tomás Faria
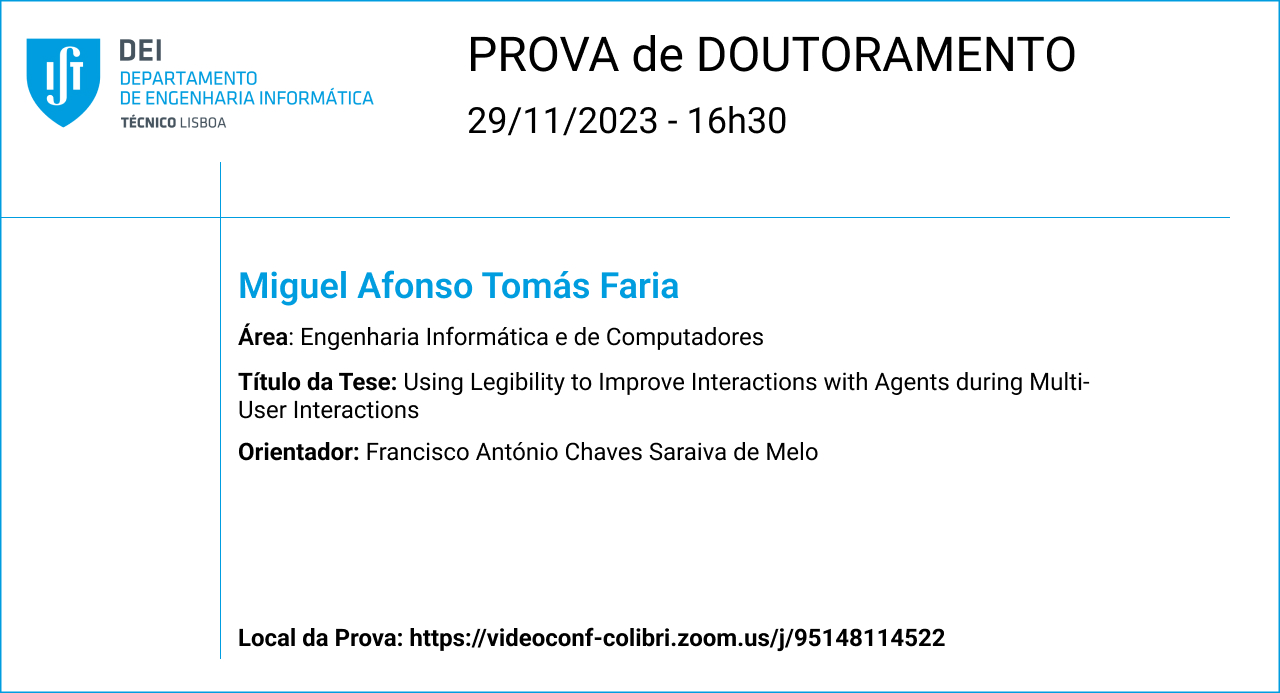
Área: Engenharia Informática e de Computadores
Título da Tese: Using Legibility to Improve Interactions with Agents during Multi-User Interactions
Local da Prova: https://videoconf-colibri.zoom.us/j/95148114522
Data: 29/11/2023
Hora: 16h30
Abstract: Clear communication is crucial for interactions between humans and robots or other artificial agents. In this thesis, we tackle the problem of improving a robot's clearness during interactions with multiple humans using legibility. Legibility measures how good a movement is in disambiguating a robot's goal from other possible goals. To explore the impact of legibility in interactions with multiple humans, we first explore how to define legibility in the context of multi-party interactions and then expand the notion of legibility beyond robotic movements to action selection and decision-making. In the first part of this thesis, we propose a definition of legibility that jointly considers the different human perspectives over the same robotic movement. Thus, legibility is defined as a function of the entire group instead of each human in particular, allowing for applications in both single-user and multi-party interactions. We also show, through a user study, that our definition of legibility for multi-party interactions improves human understanding of a robot's intentions, over the original single-user formulation. In the second part of the thesis, we take a step beyond robot motions and explore the application of legibility in the decision-making process of robots and other artificial agents. We explore the application of legibility in the context of stochastic decision-making, developing an approach for legible decision-making dubbed policy legible MDP (PoLMDP). PoLMDPs are able to handle uncertainty while remaining computationally tractable, as we show in a comparative study with other state-of-art approaches for legible decision-making. We also show, in two different studies, that our PoLMDP approach makes a robot's objectives easier to understand, both in single and multi-agent scenarios, when compared to standard MDPs.