Prova de Doutoramento do aluno Marco André Gonçalves Pinheiro
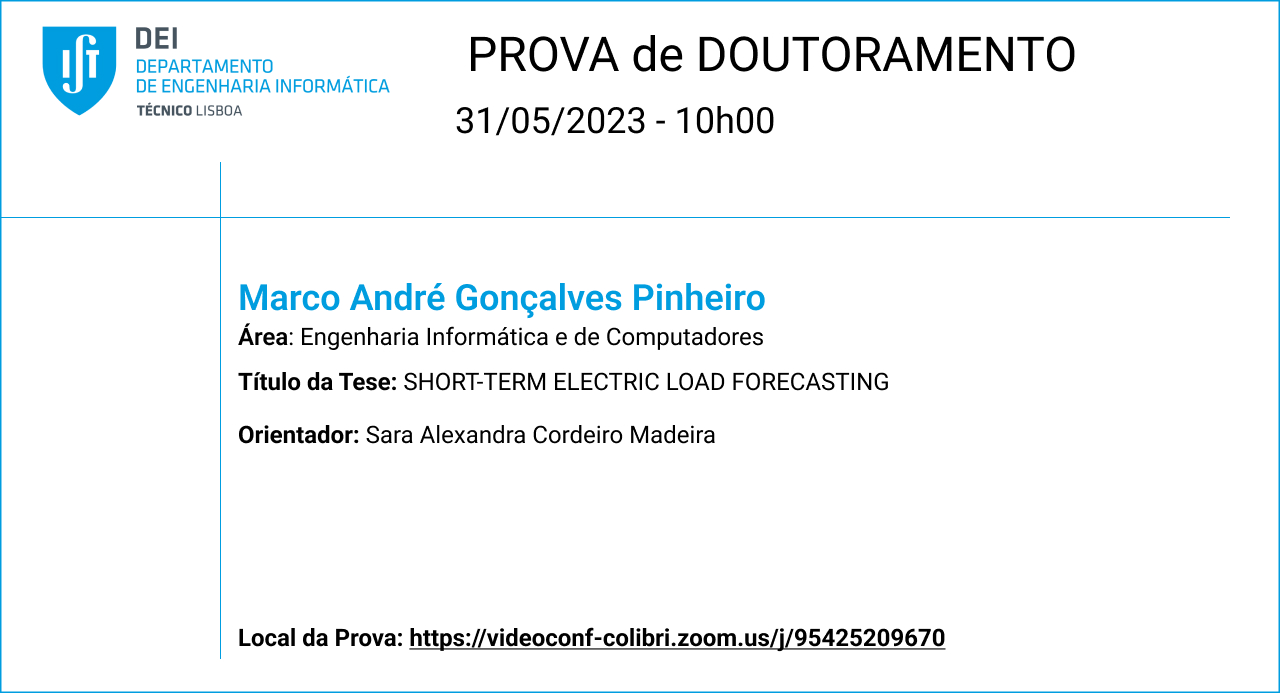
Área: Engenharia Informática e de Computadores
Título da Tese: SHORT-TERM ELECTRIC LOAD FORECASTING
Local da Prova: https://videoconf-colibri.zoom.us/j/95425209670
Data: 31/05/2023
Hora: 10h00
Abstract: Energy forecasting covers a wide range of prediction challenges in the utility industry, such as forecasting demand, generation, price, and power load over diverse time horizons and at different levels of the power grid. The short-term load forecasting in low voltage, other than at the smart meters level, has not yet been carried out in-depth compared to national/regional/building load forecasting. It is then proposed a systematic approach from the system level to the low voltage considering not only the performance of the models but also their applicability, interpretability, and reproducibility. Considering an initial benchmark model, this is compared to improved GAM (generalized additive models) enhanced by introducing new explanatory variables, reducing error by 42-47% and preserving interpretability. Additionally, an ensemble method improves accuracy for specific periods in which modeling is particularly demanding using standalone GAM models. The method is applied to the national power load and, for the first time, to all 100 000 secondary substations that integrate the Portugal power grid, rather than to tackle the few open datasets. Additionally, an appropriate data representation of power load time series, transforming them into discrete symbol sequences, is proposed and used as the base to split similar load patterns within the year, week, and special days, forming clusters. Cluster-based models are then trained with stratified sampling from the respective cluster data using the same model structure. Individual models, cluster-based models, and one-size-fits-all model are compared in terms of accuracy and applicability. This approach is used to build a live daily forecasting system called PREDIS for the Portuguese DSO whose results anticipate load peaks and network constraints. It uses a distributed system architecture which copes with both capacity and scalability challenges inherent to the storage and processing of hundreds of thousands of time series and artifacts.