Prova de Doutoramento do aluno Luís Gouveia Coutinho Sá Couto
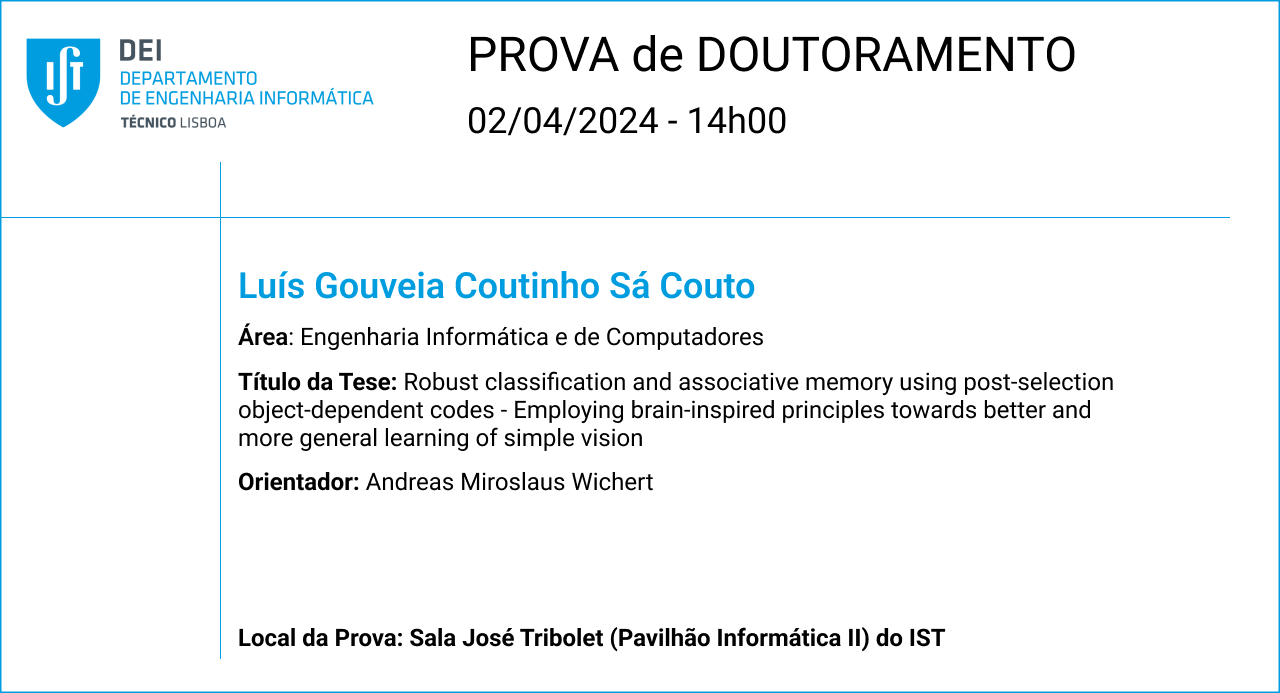
Área: Engenharia Informática e de Computadores
Título da Tese: Robust classification and associative memory using post-selection object-dependent codes - Employing brain-inspired principles towards better and more general learning of simple vision
Local da Prova: Sala José Tribolet (Pavilhão Informática II) do IST
Data: 02/04/2024
Hora: 14h00
Abstract: Deep convolutional neural networks have achieved tremendous results in a series of benchmark pattern recognition datasets, making them the current paradigm model. The phenomenon of "double-descent" is one of the most important open theoretical conundrums surrounding it. By studying it, we show that these models are very good at capturing large sets of training data and interpolating to similar test sets. Which makes them very different from humans. To illustrate this we propose a simple task that can easily be solved by humans where deep networks completely fail. The brain seems to achieve higher levels of generalization by exploiting geometric structure. We leverage the insight that the brain processes recognition with selective attention, to build a local-learning model that captures object-level geometrical in-variances. We then test multiple versions of it on computational neuroscience classification benchmarks, and show that it can solve the proposed task where current nets fail. Besides this robustness problem, unlike the brain, deep networks require tremendous amounts of energy. Binary associative memories like Willshaw's can, in theory, solve this problem provided that they deal with equally-distributed sparse inputs. However, finding encoders that can turn visual patterns into apt sparse codes has been a difficult research problem. We show how our model can produce these codes, and even further that these codes can get close to optimality when combined with a competitive learning scheme. By unlocking this memory for real data we are then able to propose a complete model of association, classification and generation entirely based on this highly-efficient, hebbian-learning-based binary network. We conclude by discussing how our approach can lay the foundation for a new framework for vision ML, provided we can extend it in the future for more complex visual patterns by including different kinds of features.
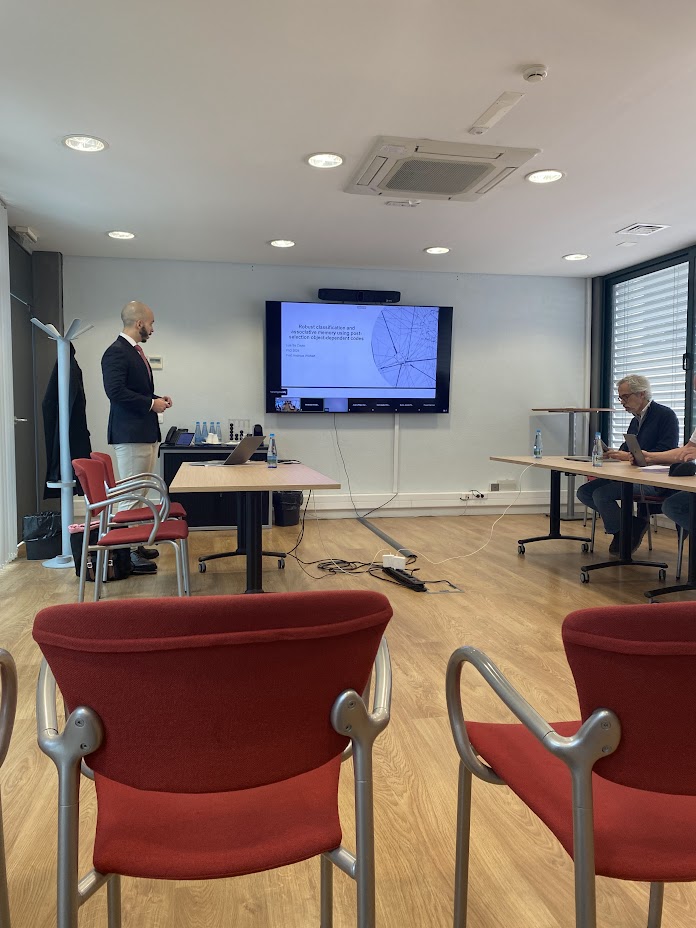
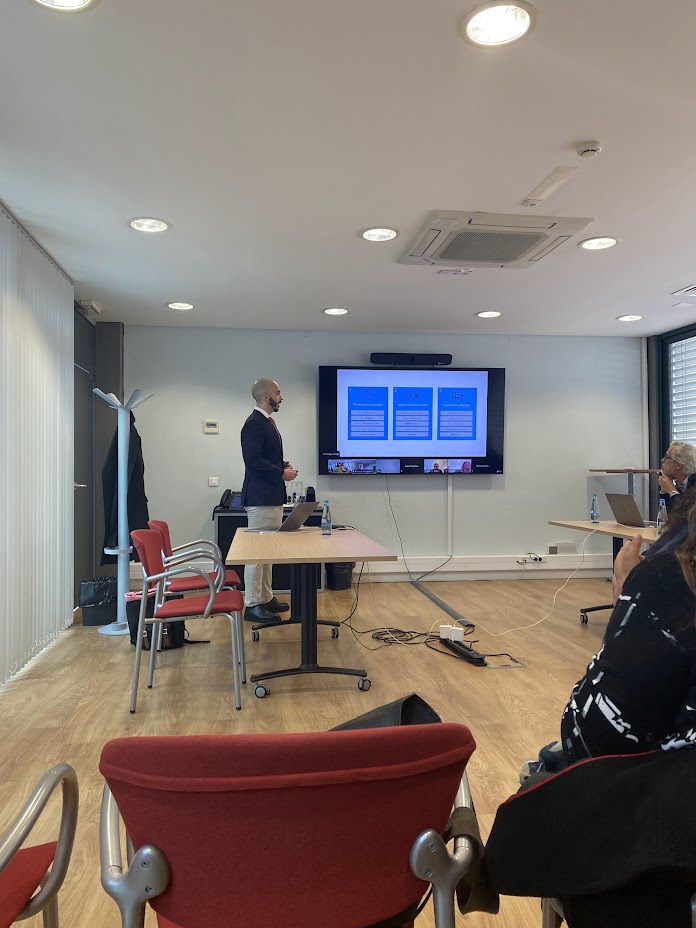
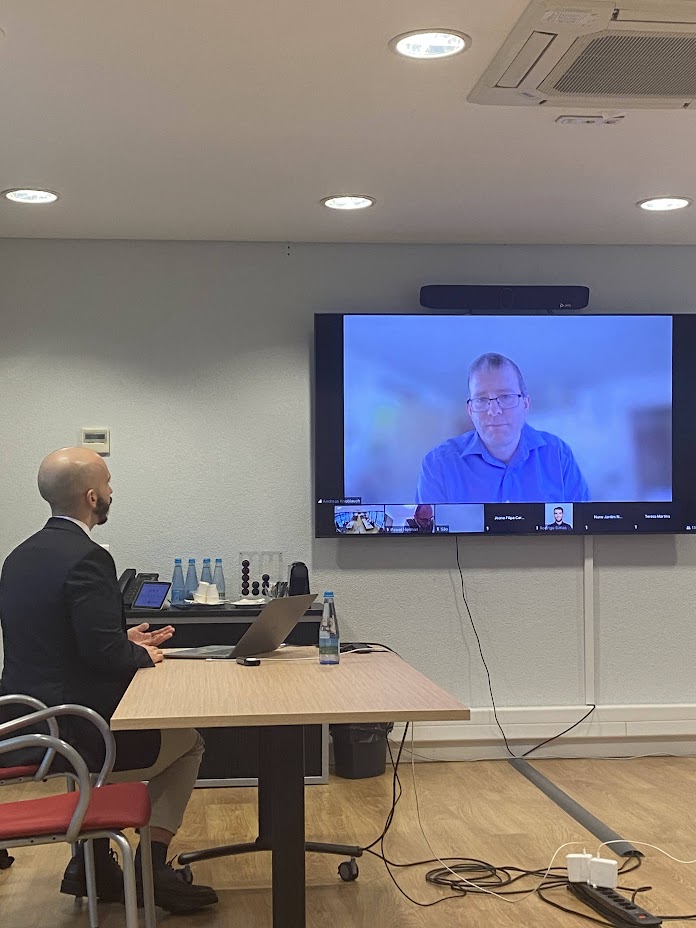
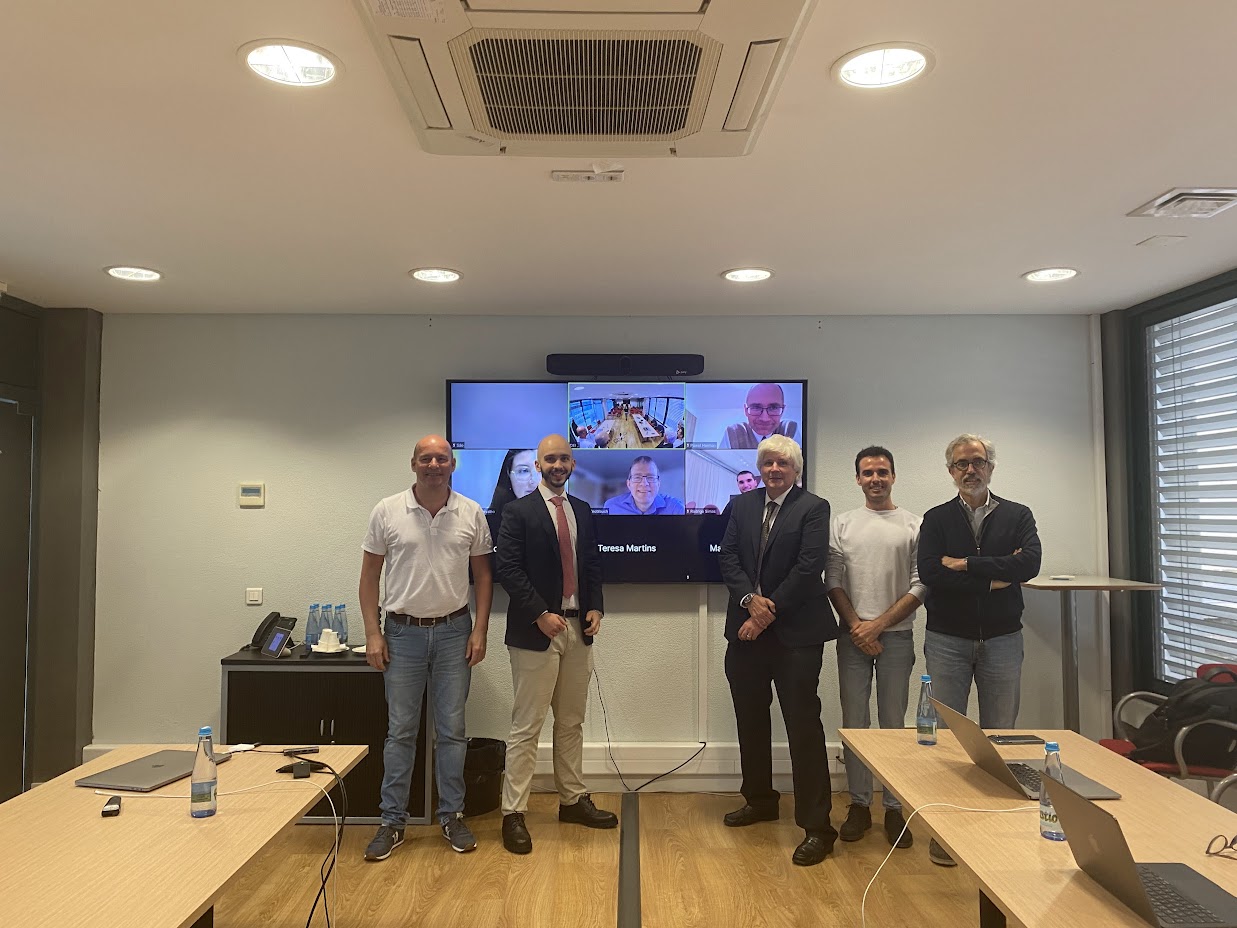