Prova de Doutoramento do aluno João Miguel Cordeiro Monteiro
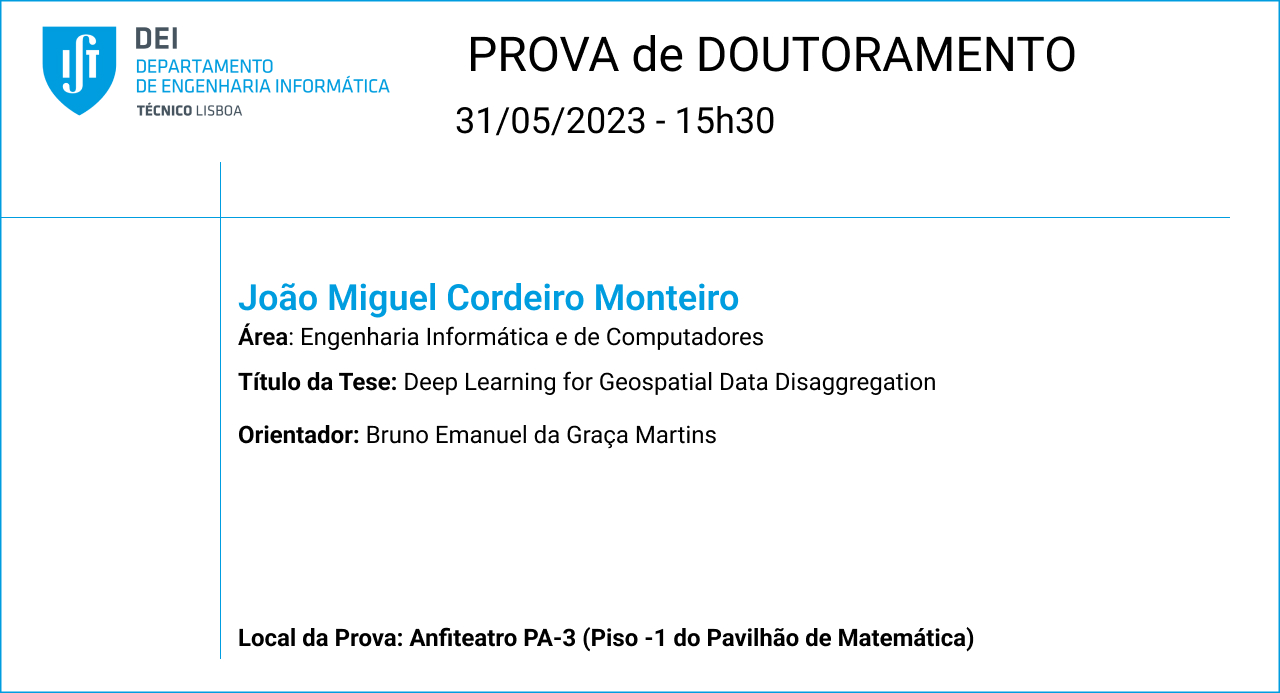
Área: Engenharia Informática e de Computadores
Título da Tese: Deep Learning for Geospatial Data Disaggregation
Local da Prova: Anfiteatro PA-3 (Piso -1 do Pavilhão de Matemática)
Data: 31/05/2023
Hora: 15h30
Abstract: My Ph.D. research project addressed the study and development of deep learning methods for geospatial data analysis, specifically focusing on the problem of spatial data disaggregation, i.e., procedures by which information at coarse spatial scales (such as districts or municipalities) is translated to finer scales, while maintaining consistency with the original dataset. A variety of previous procedures for spatial data disaggregation have been proposed, from (a) simple areal weighting, to (b) intelligent dasymetric mapping leveraging regression analysis to combine multiple sources of ancillary data. However, few previous studies have advanced deep learning methods for this particular task, despite the potential for improved results. I specifically extended previous work developed in the context of my M.Sc. thesis (i.e., a hybrid method combining pycnophylactic interpolation with regression-based dasymetric mapping), taking inspiration on current state-of-the-art neural models for processing remote sensing data (e.g., models proposed for tasks such as aerial image segmentation or super-resolution). I proposed and evaluated the application of fully-convolutional neural networks (e.g., encoder- decoder models based on the popular U-Net architecture) in different spatial data disaggregation tasks, specifically assessing the impact of different model training and regularization schemes (e.g., evaluating the impact of using different loss functions). Results show that using self-training with encoder-decoder networks to infer the dasymetric weights can outperform alternative disaggregation methods, driven by the better convergence properties achieved when applying appropriate loss functions. Moreover, a co-training strategy can achieve the best results overall, combining an encoder-decoder neural network and an ensemble of decision trees.
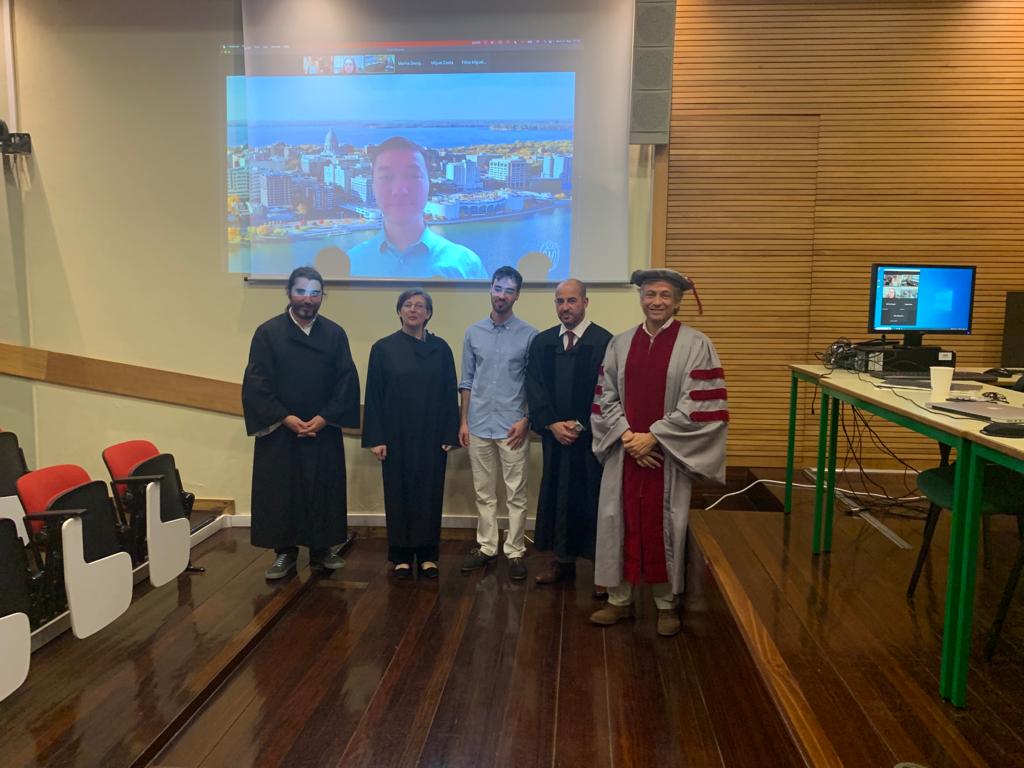