Prova de Doutoramento do aluno David António Cóias Calhas
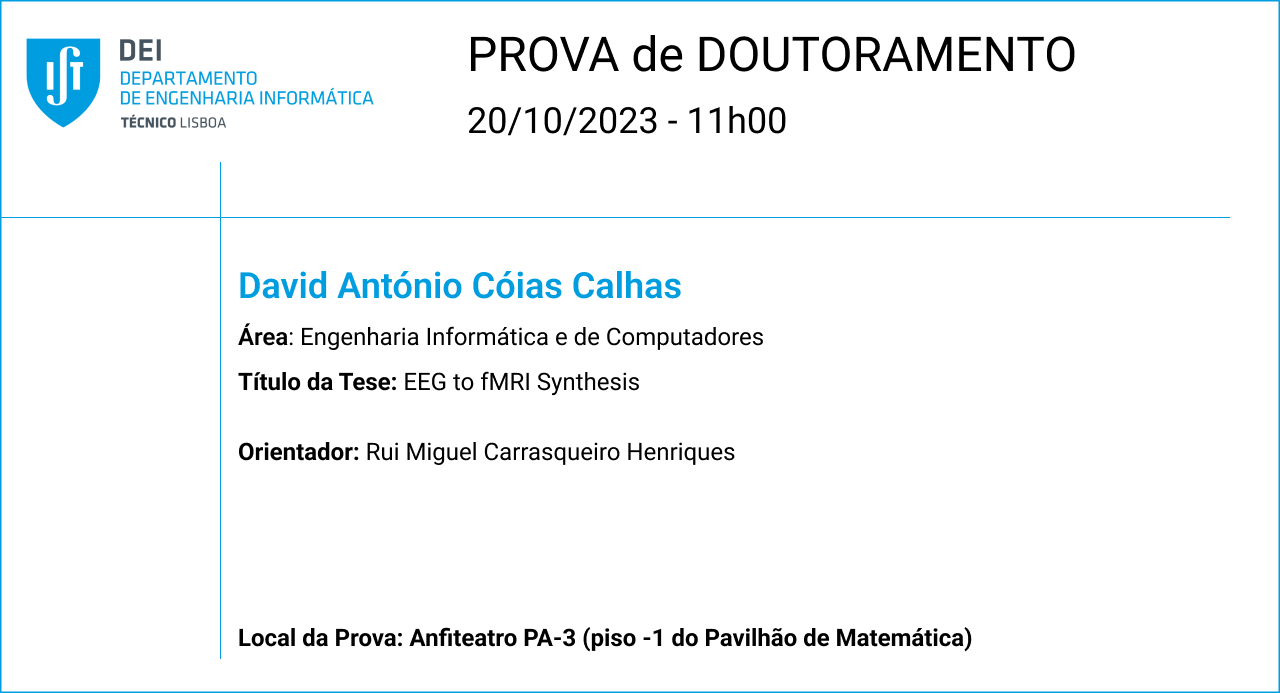
Área: Engenharia Informática e de Computadores
Título da Tese: EEG to fMRI Synthesis
Local da Prova: Anfiteatro PA-3 (piso -1 do Pavilhão de Matemática)
Data: 20/10/2023
Hora: 11h00
Abstract: Electrophysiological activity is related to haemodynamical activity, resting on the theory that the blood flows to where it is needed. As neurons fire, producing action potentials, blood irrigation is required to provide necessary molecules and remove the released toxins. Recent computational advances withheld information at high capacities of computational processing and storage, allowing a comprehensive analysis of neuroimaging records. Complementarily to Electroencephalography (EEG), the emergence of functional Magnetic Resonance Imaging (fMRI) has paved neuroscience discoveries related with haemodynamical brain activity. These modalities provide different stances on brain functions and show structurally different spatiotemporal resolutions. This work maps EEG to fMRI using neural processing principles. With such a mapping, different applications can be explored: assist ambulatory diagnostics of brain diseases; support longitudinal studies via proxy of the EEG; and increase the discriminative and interpretative power of decision support systems. In this work, we propose a automatic generation of neural architectures to give new insights to the structure of existing models and removing the human bias from the problem. To this end, simultaneous EEG and fMRI records at resting and task-based states were processed. We then converge on a neural architecture that is able to map EEG to fMRI, surpassing the state-of-the-art in this task by 13% in RMSE. Our results suggest that EEG electrode relationships (such as between Oz and PO9) are pivotal to retain information necessary for haemodynamical activity retrieval. Following, we addressed the need for uncertainty quantification in our synthesis task. The proposed solution consisted on a spectral manipulation at the output of the network, which lowers the spatial resolution of the synthesis and benefits the original versions (+0.21pp in RMSE). In the end, we show that our proposed neural network is innate at extrapolating to a classification setting, due to its shift invariant properties and spectral inspired mechanics. Using schizophrenia as a proof of concept case, we were able to prove that our synthesized view is discriminative (0.77 area under the receiver operating characteristic curve) and has a good interpretability power over this neuropsychiatric disorder.
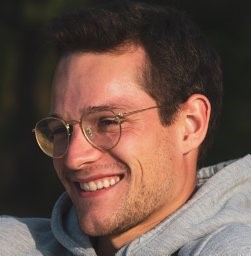