Prova de Doutoramento da aluna Taisiya Glushkova
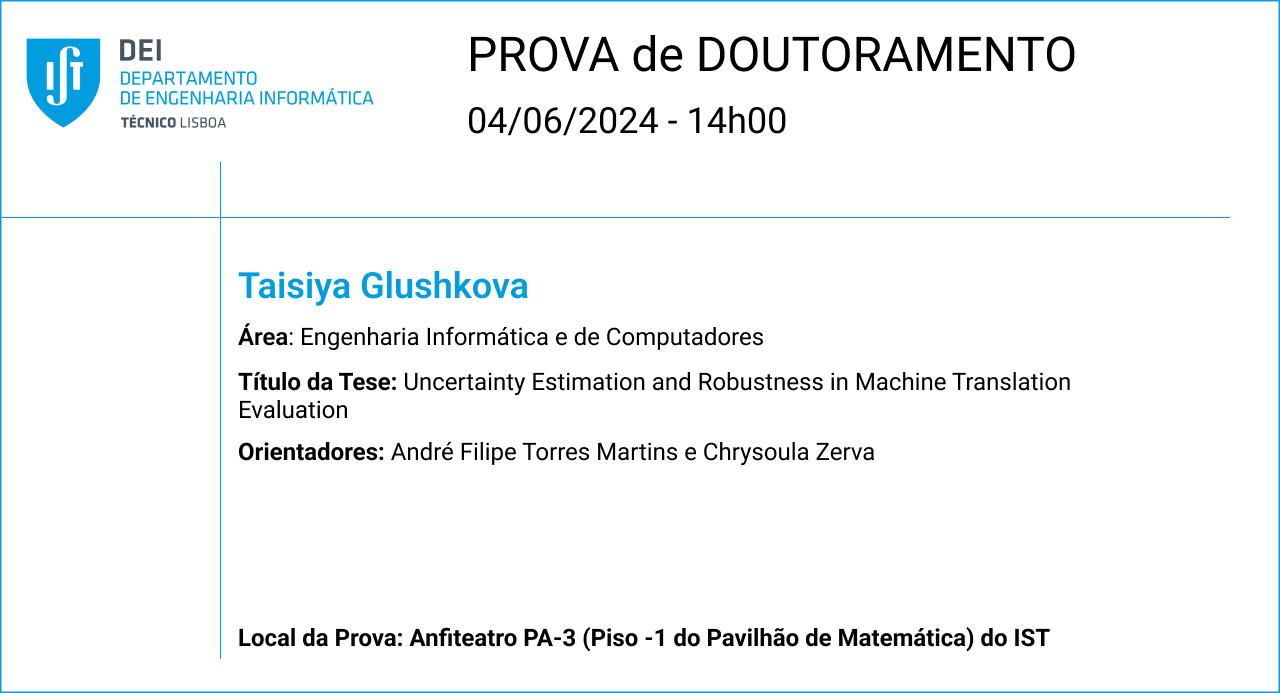
Área: Engenharia Informática e de Computadores
Título da Tese: Uncertainty Estimation and Robustness in Machine Translation Evaluation
Local da Prova: Anfiteatro PA-3 (Piso -1 do Pavilhão de Matemática) do IST
Data: 04/06/2024
Hora: 14h00
Abstract: This thesis addresses the challenge of enhancing machine translation (MT) evaluation methods, with a strong focus on precision, trustworthiness and interpretability of predictions, aiming to increase the robustness of the underlying models. The work unfolds through three main stages, each contributing to the refinement of neural-based MT evaluation models. First we focus on enhancing trainable neural-based MT evaluation metrics by incorporating a measure of confidence in their quality predictions. We propose a straightforward way for developing uncertainty-aware quality estimation models by representing quality as a distribution rather than a single value. We compare two well-established uncertainty estimation techniques, Monte Carlo (MC) dropout and deep ensembles, in combination with the COMET metric. These approaches allow for flexible use of the same system with varying numbers of references, with confidence intervals narrowing as more references are added, leading to enhanced confidence in MT evaluation with increased information. Our evaluations demonstrate that the uncertainty-aware systems exhibit better calibration concerning human direct assessments (DA), multi-dimensional quality metric scores (MQM), and human translation error rates (HTER) compared to a simple baseline. Moreover, the uncertainty-aware evaluation systems enable a promising quality estimation use case for automatically detecting low-quality translations using a risk-based criterion. The second stage delves deeper into uncertainty quantification, aiming to overcome some of the identified limitations, such as their high inference or training time costs and inability to distinguish between different sources of uncertainty. We further explore more advanced and sophisticated approaches that offer greater efficiency and accuracy: direct uncertainty prediction, leveraging supervision over quality prediction errors; heteroscedastic regression, estimating input-dependent aleatoric uncertainty and combinable with MC dropout; and divergence minimization, estimating uncertainty from annotator disagreements in the presence of multiple annotations for the same example. These methods represent a significant step forward in accurately targeting specific types of uncertainty, addressing aleatoric uncertainty through heteroscedastic regression and divergence minimization, and epistemic uncertainty through direct uncertainty prediction. Finally, we turn to the quest for more interpretable and robust MT evaluation methods. We seek to address issues related to unreliability in detecting certain critical errors, such as deviations in entities and numbers. By combining traditional evaluation metrics, like BLEU and CHRF, which measure lexical overlap between translation hypotheses and human references, and therefore are sensitive to such deviations, with neural-based metrics, we strengthen their capability to penalize translations with specific problematic phenomena. We show that by using additional information during training, such as sentence-level features, word-level tags and word factors, higher correlation with human judgments and substantial gains on recent challenge sets across various language pairs can be achieved. To foster future research, we have made all resources publicly available, including code, data, and pretrained models. Our work aims to provide a solid foundation for advancing the state-of-the-art in MT evaluation, paving the way for more reliable and interpretable evaluation methods, ultimately benefiting the field of natural language processing (NLP) and machine translation.