Prova de Doutoramento da aluna Carla Andreia Nibau Guerra de Azevedo
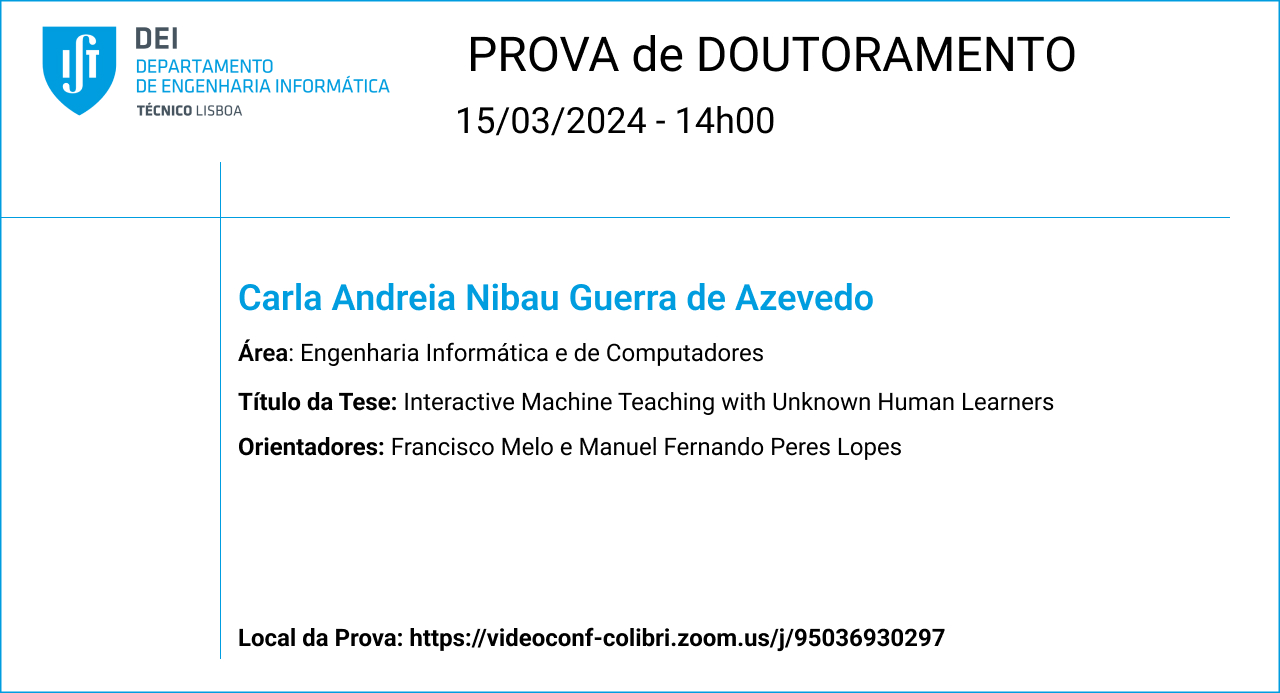
Área: Engenharia Informática e de Computadores
Título da Tese: Interactive Machine Teaching with Unknown Human Learners
Local da Prova: https://videoconf-colibri.zoom.us/j/95036930297
Data: 15/03/2024
Hora: 14h00
Abstract: Machine teaching systems assume that the learner is perfectly known, which is seldom true. There is, thus, an unavoidable mismatch between how the machine teaching systems model the learners and the learners themselves. In this thesis, we propose the use of interactivity as the means to mitigate the negative impact of having imperfect knowledge about the learners. This leads us to our main research question: - How can interactivity be used in machine teaching systems to improve the learning performance of students? To address this question we assume the teacher captures the learner through a model that can be fine-tuned by asking questions to the learner. With a more realistic model of the learner, the system can select teaching samples that are better tailored for them and make learning more efficient. Our main contributions include the proposal and/or evaluation of three interactive teaching approaches. The first two teach a Bayesian inference task to single and multiple learners, respectively. We run three user studies where we investigate the impact of having imperfect knowledge about the learners in real-world scenarios and how interactivity can help overcome it. In multiple-learner settings we explored two ways to address the class -- as a whole or dividing it into partitions according to the learner's priors. Finally, we propose a Feature Importance-based Teaching (FIT) approach that teaches classification tasks. It uses feedback on the learner's feature weights to estimate the learner's prior knowledge (i.e. the samples it previously saw) and then adaptively selects the samples that minimize the expected future error. We tested the proposed approach in simulated and real-world environments within two different domains: 1) food literacy and (2) breast cancer image analysis. We also simulated with multi-learner settings. All the results confirmed that considering interactivity in the teaching process outperforms the alternatives considered.
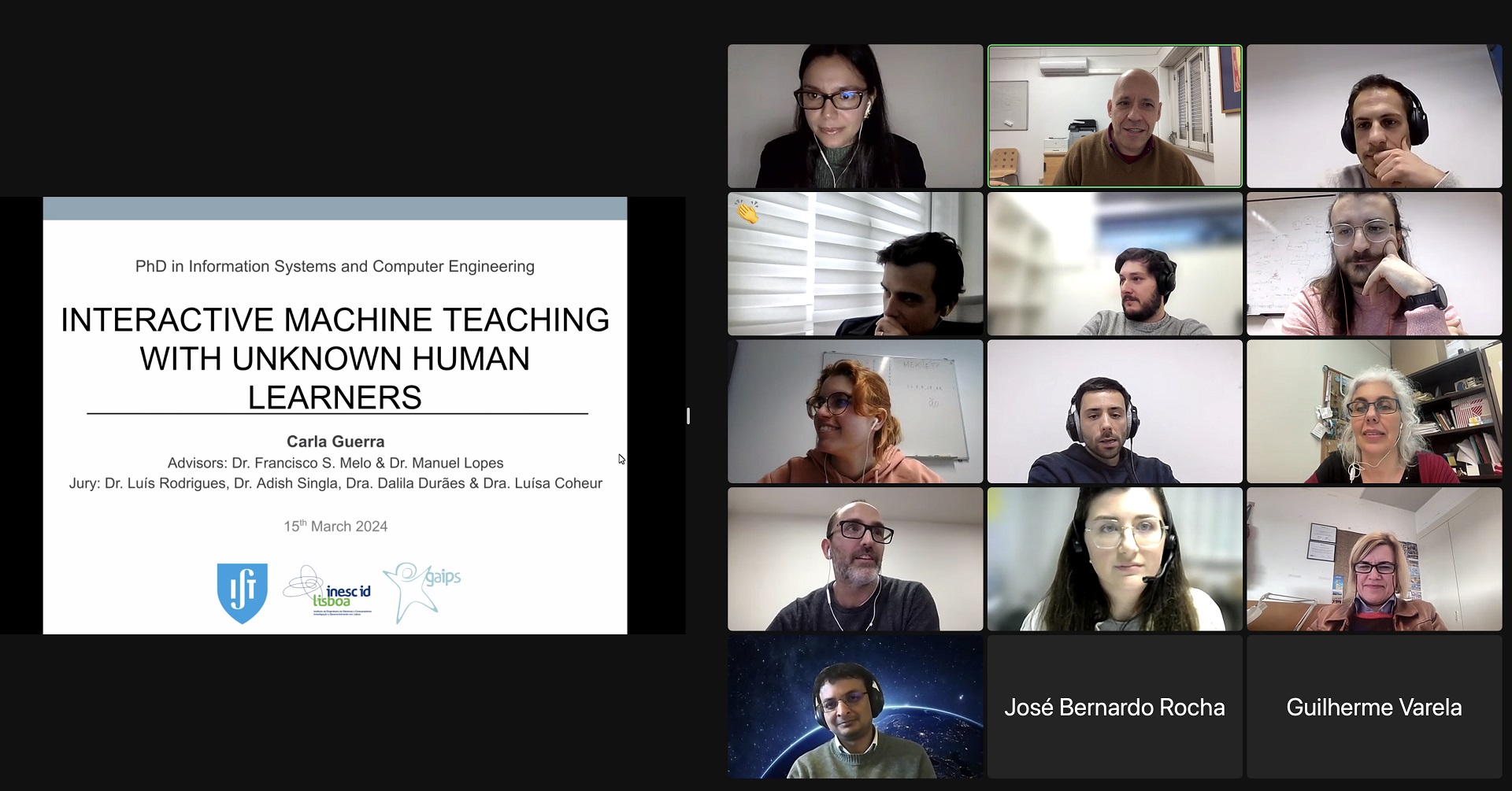