Wednesdays@DEI: Talks, 20-09-2023
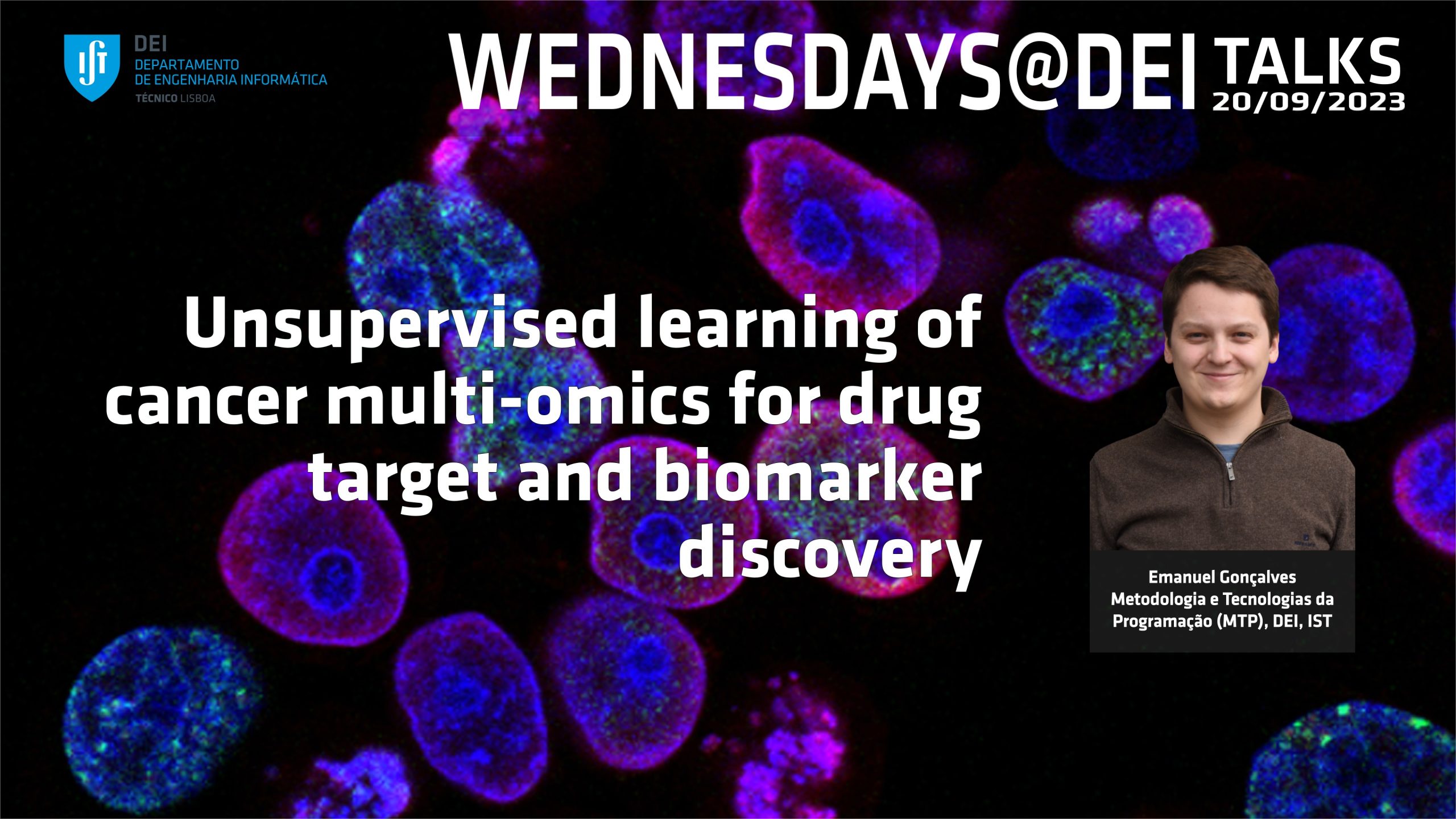
Autor e vínculos: Professor Emanuel Gonçalves; Área científica: Metodologia e Tecnologias da Programação (MTP)
Título: Unsupervised learning of cancer multi-omics for drug target and biomarker discovery
Abstract: Comprehensive analyses of cancer cells through systematic pharmacological and CRISPR-based genetic screening have unveiled numerous potential actionable dependencies. Surprisingly, genetic alterations can account for less than 20% of these cancer dependencies. This underscores the urgency to develop machine learning models that can seamlessly integrate diverse datasets beyond genomics, encompassing molecular and phenotypic profiling of cancer cells.
We illustrate the effectiveness of unsupervised learning to robustly integrate sparse and highly heterogeneous multi-omic datasets, spanning genomics, proteomics, and drug response screens across hundreds of cancer cell lines. Model interpretation sheds light on the molecular processes that dominate cellular variability in cancer, spotlighting the critical role of the epithelial-to-mesenchymal transition (EMT) – a pivotal process in cancer drug resistance and metastasis.
Our study serves as a compelling demonstration of unsupervised learning's capacity to harmonize diverse data sources and construct resilient models for elucidating intricate cancer phenotypes, ultimately advancing our understanding of cancer dependencies and potential therapeutic targets.