Wednesdays@DEI: Talks, 07-06-2023
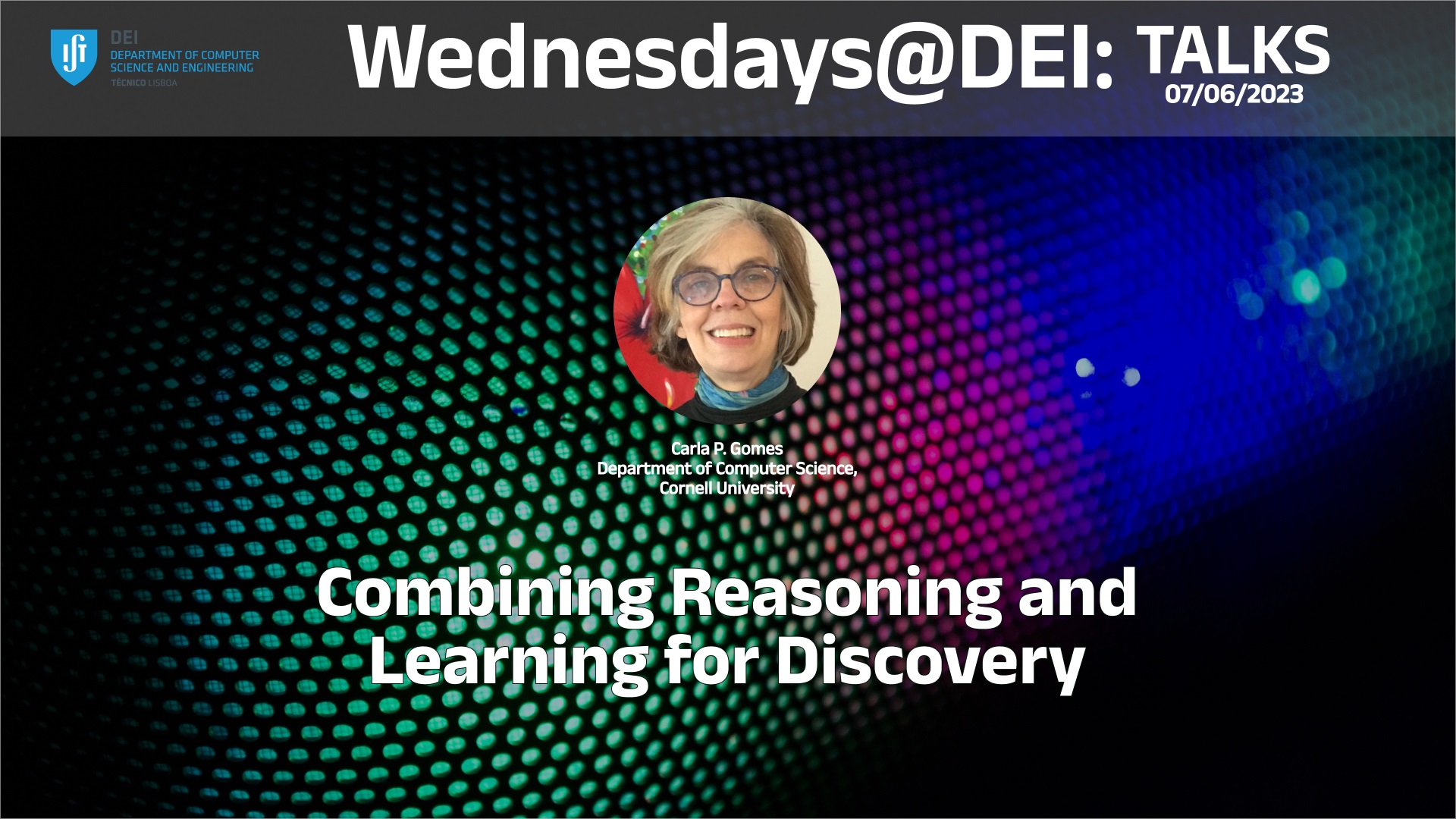
Autor e vínculos: Professora Carla P. Gomes, Cornell University, Department of Computer Science
Título: Combining Reasoning and Learning for Discovery
Abstract: Artificial Intelligence (AI) is a rapidly advancing field inspired by human intelligence. AI systems are now performing at human and even superhuman levels on various tasks, such as image identification, face and speech recognition, and chatbots such as chatGPT. The tremendous AI progress that we have witnessed in the last decade has been largely driven by deep learning advances and heavily hinges on the availability of large, annotated datasets to supervise model training. However, often we only have access to small datasets and incomplete data. We amplify a few data examples with human intuitions and detailed reasoning from first principles and prior knowledge for discovery. I will talk about our work on AI for accelerating the discovery for new solar fuels materials, which has been featured in Nature Machine Intelligence, in a cover article entitled, Automating crystal-structure phase mapping by combining deep learning with constraint reasoning [1]. In this work, we propose an approach called Deep Reasoning Networks (DRNets), which seamlessly integrates deep learning and reasoning via an interpretable latent space for incorporating prior knowledge. and tackling challenging problems. DRNets requires only modest amounts of (unlabeled) data, in sharp contrast to standard deep learning approaches. DRNets reach super-human performance for crystal-structure phase mapping, a core, long-standing challenge in materials science, enabling the discovery of solar-fuels materials. DRNets provide a general framework for integrating deep learning and reasoning for tackling challenging problems. For an intuitive demonstration of our approach, using a simpler domain, we also solve variants of the Sudoku problem. The article DRNets can solve Sudoku, speed scientific discovery [2] provides a perspective for a general audience about DRNets. DRNets is part of SARA, the Scientific Reasoning Agent for materials discovery [3]. Finally, I will also talk about the effectiveness of a novel curriculum learning with restarts strategy to boost a reinforcement learning framework [4]. We show how such a strategy is characterized by left heavy-tails and can outperform specialized solvers for Sokoban, a prototypical AI planning problem.