Wednesdays@DEI: Talks, 22-02-2023
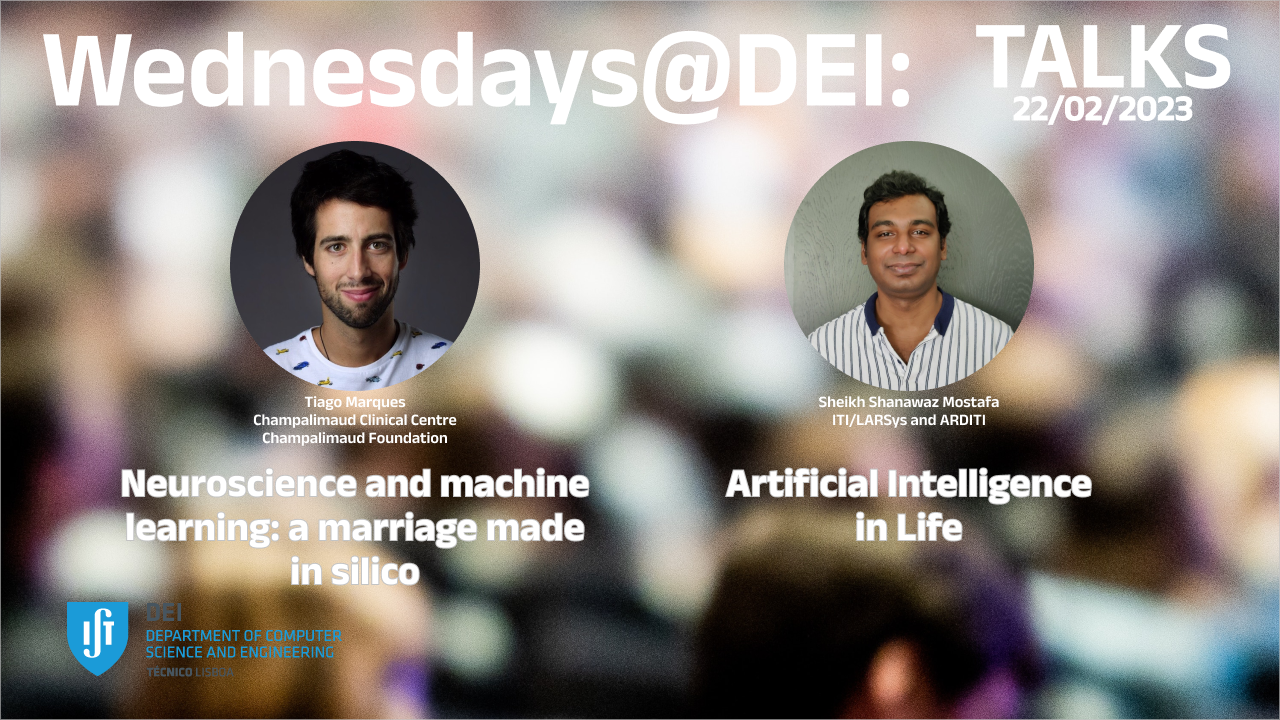
Author: Tiago Marques, Champalimaud Clinical Centre, Champalimaud Foundation
Title: Neuroscience and machine learning: a marriage made in silico
Abstract: In neuroscience research, computational models are used as tools for understanding brain function and behavior. These models typically have a narrow domain and small number of parameters, failing to explain the complexity of multi-scale neuronal systems. Machine learning research, on the other hand, often uses weakly constrained models with millions of parameters to solve tasks that humans execute more robustly. In this talk, I review some of my recent work exploring the synergies between neuroscience and machine learning. I start by exploring how artificial neural networks (ANNs) have been successfully used as computational models of visual processing in the brain and how their brain similarity relates to accuracy and robustness in computer vision tasks. Then, I present a novel hybrid ANN that I developed, the VOneNet, inspired and constrained by neurobiology, that improves in challenging computer vision problems by being more robust to a wide range of image perturbations. This has important implications for real-world applications suggesting that biological intelligence still has a lot of untapped potential to contribute to artificial systems. Together these projects show that machine learning and neuroscience can benefit from a virtuous cycle, wherein research in each reinforces the ability and our understanding of the other.
Bio: How does hierarchical processing in the brain give rise to sensory perception, and can we use this understanding to develop more human-like machine learning algorithms? Answering these questions has been the focus of Tiago's research during most of his career. After a Masters in Physics Engineering at Instituto Superior Técnico, he first encountered the problem of visual perception during his PhD at the Champalimaud Foundation, where he studied visual cortical processing in the mouse. Under the supervision of Leopoldo Petreanu, Tiago developed a motion discrimination task for mice and established a causal link between activity in the primary visual cortex (V1) and motion perception. Following that project, he studied the functional organization of cortical feedback and showed that feedback inputs in V1 relay contextual information to matching retinotopic regions in an organized manner. In 2019, Tiago joined the lab of Prof. James DiCarlo at MIT to continue his training as a PhRMA Foundation Postdoc Fellow, where he used artificial neural networks (ANNs) as models of primate vision. Relying on available neuronal datasets, Tiago developed novel benchmarks to evaluate how well different ANNs match primate V1, observing that models that better align with early vision are also better at predicting object recognition behavior. Inspired by this relationship, Tiago went on to build a novel ANN architecture constrained by a neuroscientific model of vision. The resulting model family, the VOneNet, is not only more aligned with visual processing in the brain, but is also more robust to adversarial attacks. In late 2022, Tiago returned to the Champalimaud Foundation, where he is co-leading a project to bring Artificial Intelligence and Augmented Reality technologies to the clinic to allow live, digital, non-invasive, image-guided precision surgery for breast cancer. By working simultaneously in fundamental and translational science, Tiago wishes to accomplish his goal of bridging knowledge from neuroscience to machine learning models, and deploying these in real-world applications.
Author: Sheikh Shanawaz Mostafa, Interactive Technologies Institute/ LARSys and ARDITI
Title: Artificial Intelligence in Life
Abstract: Artificial intelligence (AI) has increasingly become an integral part of our daily lives, spanning diverse areas ranging from sleep to air traffic control. Given that sleep constitutes one-third of our lives, machine learning (ML) algorithms, a subset of AI, have been developed to detect and diagnose sleep-related problems, such as apnea, as well as to create intelligent devices that monitor sleep quality using oxygen saturation (SpO2), electrocardiogram (ECG), and electroencephalogram (EEG). ML has also found application in sports research, where it can predict injuries within one-day, seven days, and one-month windows, and prevent non-contact injuries among professional football players by analysing GPS data and identifying the risk factors that could lead to injuries. Combining ML with robotics has led to the development of highly advanced and intuitive robotic prosthetics that mimic natural limb movements and provide greater independence and mobility for people with limb loss or differences. To this end, the force estimator, which uses electromyography (EMG) and ML, was developed. EMG signals are also being utilized in the development of voiceless sound recognition systems with potential applications in silent speech interfaces and even vocal coaching and therapy. Natural language processing (NLP) and ML have enabled AI to more effectively analyse and interpret the emotional content and sentiment of language, leading to the development of applications such as sentiment analysis with personalized reports for restaurant owners, and personalized recommendations for improvement. Additionally, ML algorithms can be used to forecast wind speed and direction over very short ranges, a critical factor in air traffic operations, which allows for more accurate and efficient scheduling and routing of flights. In the search and optimization of machine learning models, bio-inspired techniques such as genetic algorithms and swarm optimization can be utilized to develop accurate and efficient predictive models in various fields, such as healthcare and natural language processing. Finally, the acceleration of ML models is an essential component of their deployment, with field-programmable gate arrays (FPGAs) implemented to achieve high-throughput ANN and CNN models.
Bio: Sheikh Shanawaz Mostafa is currently a postdoctoral researcher in ARDITI and ITI/LARSys; he received a Ph.D. Degree from Instituto Superior Técnico of the University of Lisbon, Portugal, in 2020. He has a multi-disciplinary education; B.Sc. Eng. Degree in Electronics & Communication, M.Sc. in Biomedical, and Ph.D. in Electrical and Computer. His research varies from biomedical to agriculture, and natural language processing, with the hardware implementation and the core being machine learning and artificial intelligence. He worked on several research projects with companies and academic settings.